Bank Fraud detection with automatic learning
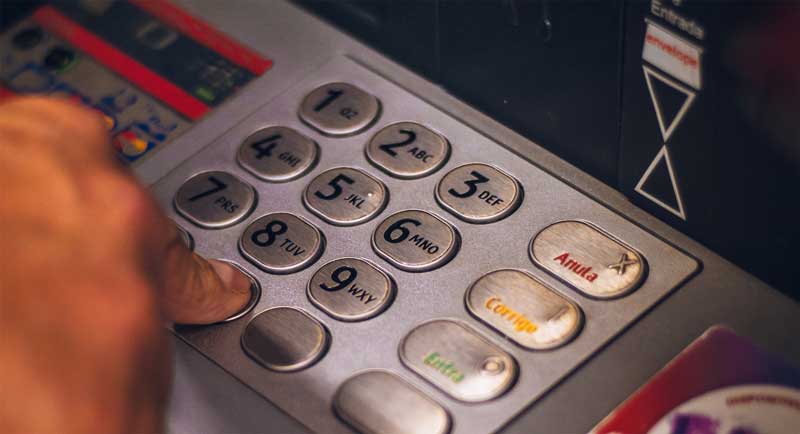
The financial sector is currently immersed in a fight against bank fraud, with this being one of its biggest challenges. Spanish banking saw an increase of 17.7% in 2018 in claims for improper transactions or charges compared to the previous year and in 2017 alone there were over 123,064 on-line fraud incidents against companies and individuals.
The banking system is confronting the battle against fraud from a technological point of view. It is currently in the midst of a digitisation process and, with investments of around 4,000 million euros per year, it is putting its efforts into adoption of new technologies such as Big Data and Artificial Intelligence. These technologies are intended to improve and automate various processes, including detection and management of fraud.
At /bluetab we are undertaking a variety of initiatives within the technological framework of Big Data and Artificial Intelligence in the financial sector. Within the framework of our “Advanced Analytics & Machine Learning” initiatives, we are currently collaborating on Security and Fraud projects where, through the use of Big Data and Artificial Intelligence, we are able to help our clients create more accurate predictive models.
So, how can automatic learning help prevent bank fraud?. Focusing on collaborations within the fraud area, /bluetab addresses these types of initiatives based on a series of transfers identified as fraud and a data set with user sessions in electronic banking. The challenge is to generate a model that can predict when a session may be fraudulent by targeting the false positives and negatives that the model may produce.
Understanding the business and the data is critical to successful modelling.
In overcoming these kinds of technological challenges, we have noted how the use of a methodology is of vital importance in addressing these challenges. At /bluetab we make use of an in-house, ad-hoc adaptation for Banking of the CRISP-DM methodology in which we distinguish the following phases:
- Understanding the business
- Understanding the data
- Data quality
- Construction of intelligent predictors
- Modelling
We believe that in On-line Fraud detection projects understanding the business and the data is of great importance for proper modelling. Good data analysis lets us observe how these are related to the target variable (fraud), as well as other statistical aspects (data distribution, search for outliers, etc.) which are of no less importance. You can note the presence in these analyses of variables with great predictive capacity, which we call “diamond variables”. Attributes such as the number of visits to the website, the device used for connection, the operating system or the browser used for the session (among others) are usually strongly related to bank fraud. In addition, the study of these variables shows that, individually, they can cover over 90% of fraudulent transactions. That is, analysing and understanding the business and the data enables you to evaluate the best way of approaching a solution without getting lost in a sea of data.
Once you have the understanding of the business and the data and after having obtained those variables with greater predictive power, it is essential to have tools and processes that ensure the quality of those variables. Training the predictive models with reliable variables and historical data is indispensable. Training with low-quality variables could lead to erratic models with great impacts within the business.
After ensuring the reliability of the selected predictor variables, the next step is to construct intelligent predictor variables. Even though these variables, selected in the previous steps, have a strong relationship with the variable to be predicted (target), they can result in certain problems in behaviour when modelling, which is why data preparation is necessary. This data preparation involves making certain adaptations to the variables to be used within the algorithm, such as the handling of nulls or of categorical variables. Additionally, proper handling of the outliers identified in the previous steps must be performed to avoid including information that could distort the model.
With the aim of “tuning” the result, it is similarly of vital importance to apply various transformations to the variables to improve the model’s predictive value. Basic mathematical transformations such as exponential, logarithmic or standardisation, together with more complex transformations such as WoE, make it possible to substantially improve the quality of the predictive models thanks to the use of more highly processed variables, facilitating the task of the model.
Finally, the modelling stage focuses on confronting different types of algorithms with different hyperparameter configurations to get to the model that generates the best prediction. This is where tools such as Spark help to a great extent, by being able to carry out training of different algorithms and configurations quickly, thanks to distributed programming.
For sustainability of your application and to avoid model obsolescence, this methodology needs to be followed monthly in each use case and more frequently when dealing with an initiative such as bank fraud. This is because new forms of fraud may arise that are not covered by the trained models. This means it is important to understand and to select the variables with which to retrain the models so that they do not become obsolete over time, which could seriously harm the business.
In summary, a good working methodology is vital when addressing problems within the world of Artificial Intelligence and Advanced Analytics, with phases for understanding the business and the data being essential. Having specialised internal tools to enable these types of projects to be executed in just a few weeks is now a must, to generate quick wins for our clients and their business.